Using PostgreSQL Arrays The Right Way
At Heap, we lean on PostgreSQL for most of the backend heavy lifting.[1] each event as an hstore blob, and we keep a PostgreSQL array of events done by each user we track, sorted by time. Hstore lets us attach properties to events in a flexible way, and arrays of events give us great performance, especially for funnel queries, in which we compute the drop off between different steps in a conversion funnel.
In this post, we’ll take a look at a PostgreSQL function that unexpectedly hung on large inputs and rewrite it in an efficient, idiomatic way.
Your first instinct might be to treat arrays in PostgreSQL like their analogues in C-based languages. You might be used to manipulating data via array positions or slices. Be careful not to think this way in PostgreSQL, especially if your array type is variable length, e.g. json, text, or hstore. If you’re accessing a PostgreSQL array via its positions, you’re in for an unexpected performance blowup.
This came up a few weeks ago at Heap. We keep an array of events for each user tracked by Heap, in which we represent each event with an hstore datum. We have an import pipeline that appends new events to the right arrays. In order to make this import pipeline idempotent, we give each event an event_id
entry, and we run our event arrays through a utility function that squashes duplicates. If we want to update the properties attached to an event, we just dump a new event into the pipeline with the same event_id
.
So, we need a utility function that takes an array of hstores, and, if two events have the same event_id
, it should take the one that occurs later in the array. An initial attempt to write this function looked like this:
This works, but it blows up for large inputs. It’s quadratic, and it takes almost 40 seconds for an input array of 100k elements!
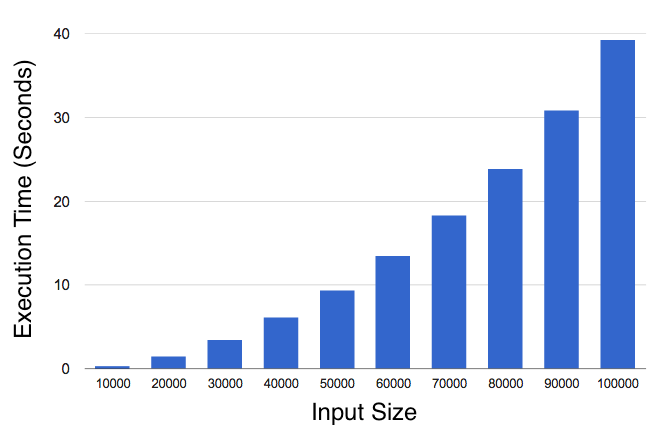
Test queries were timed on a macbook pro with a 2.4GHz i7 and 16 GB of ram and generated with this script: https://gist.github.com/drob/9180760.
What’s going on here? The issue is that PostgreSQL stores an array of hstores as an array of values, not an array of pointers to values. That is, an array of three hstores looks something like
{“event\_id=>1,data=>foo”, “event\_id=>2,data=>bar”, “event\_id=>3,data=>baz”}
under the hood, as opposed to
{[pointer], [pointer], [pointer]}
For types that are variable length, e.g. hstores, json blobs, varchars, or text fields, PostgreSQL has to scan the array to find the Nth element. That is, to evaluate events[2]
, PostgreSQL parses events from the left until it hits the second entry. Then, for events[3]
, it re-scans from the first index all over again until it hits the third entry! So, evaluating events[sub]
is O(sub), and evaluating events[sub]
for each index in the array is O(N2), where N is the length of the array.
PostgreSQL could be smarter about caching intermediate parse results, or it could parse the array once in a context like this. The real answer is for arrays of variable-length elements to be implemented with pointers to values, so that we can always evaluate events[i]
in constant time.
Even so, we shouldn’t rely on PostgreSQL handling this well, since this is not an idiomatic query. Instead of generate_subscripts
, we can use unnest
, which parses an array and returns a set of entries. This way, we never have to explicitly index into the array.
This is efficient, and it takes an amount of time that’s linear in the size of the input array. It takes about a half a second for an input of 100k elements, compared to 40 seconds with the previous implementation.
This does what we want:
Parse the array once, with
unnest
.Partition by
event_id
.Take the last occurrence for each
event_id
.Sort by input index.
Lesson learned: if you find yourself accessing positions in a PostgreSQL array, consider unnest instead.
We like to avoid stubbing our toes. Have any feedback or other PostgreSQL tips? Shoot us a note @heap.
[1] In particular, we use a lovely tool called Citus Data. More on that in another blog post!