Data-Informed
How to choose the right structure for your data analytics team
These days, you can’t just make critical business decisions based on gut instinct alone. With today’s pace and competition, you need to rely on a host of different elements to drive strong decision-making. And your most powerful weapon? Digital analytics.
Digital analytics is no longer a nice-to-have. With the volume of data that now surrounds us on a daily basis, digital analytics acts as your guiding compass; providing you with valuable insights into what's working well and what isn't, allowing you to optimize your products, understand your customers, and ultimately increase your ROI.
But let's face it, building a successful data culture can be overwhelming. It's not just about collecting data–you need a well-structured analytics team to make sense of it all, too. Enter one of the most important individuals you’ll need to assign: an administrator role. This person will be responsible for making sure the right people have access to the right data, while also ensuring that sensitive information stays safe and secure.
In this post, we’ll explore the three common structures of data analytics teams: centralized, hub and spoke, and decentralized.
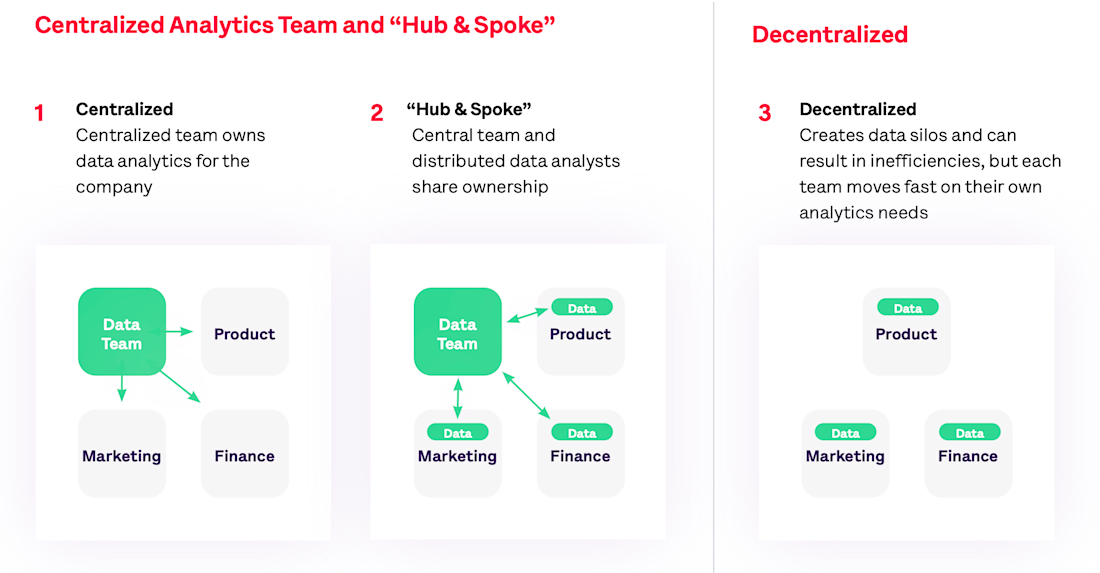
We’ll cover all the details you need, including the different roles and responsibilities within each model, as well as best practices for creating an effective team that supports your organization's goals. By the time you're done reading, you'll be well-equipped to build the analytics team of your dreams.
Let’s get started.
1 - Centralized model
What is it?
A centralized model is pretty simple: it keeps things central. Instead of having a bunch of separate teams doing their own analytics, all analytics operations are managed and executed by one team. They handle everything from managing data to analyzing it, and they do it for your entire organization.
A key benefit of a centralized model is the consistency it offers. With a centralized model, everyone in your business has access to the same data sources. What’s more, data analysis is standardized across the board. This is especially helpful if you have a big business with complex data needs, as it helps improve accuracy and decision-making.
Who uses it?
Typically, larger mid-market companies and enterprises are the ones to adopt a centralized approach. It makes sense for them because one team owns the entire analytics tech stack, including all analytics applications and data warehouses.
But here’s the thing: you need the right people on your analytics team to make it work.
So here are some recommendations for roles and responsibilities you should consider when putting your team together:

2 - Hub and Spoke
What is it?
A hub and spoke model is an easy way to balance things out between a centralized model and a decentralized model. It’s where one team of data administrators and analytics experts oversee everything, but each business function (or “spoke”) gets to have their own data analysts who can move quickly on their own. This might be functions like product, marketing, or finance.
The best thing about a hub and spoke model is that it makes sure everyone in your organization is on the same page. People, process, and technology are all aligned. The idea behind this model is to make sure analytics are right there with the decision-makers, so you can grow and evolve faster.
Who uses it?
The hub and spoke model is not just for big enterprises like the centralized model. This one applies to any mid-market to enterprise organization that wants both agility and consistency when it comes to data analytics.
So if you’re thinking of using the hub and spoke model, we recommend including these roles and responsibilities on your team:
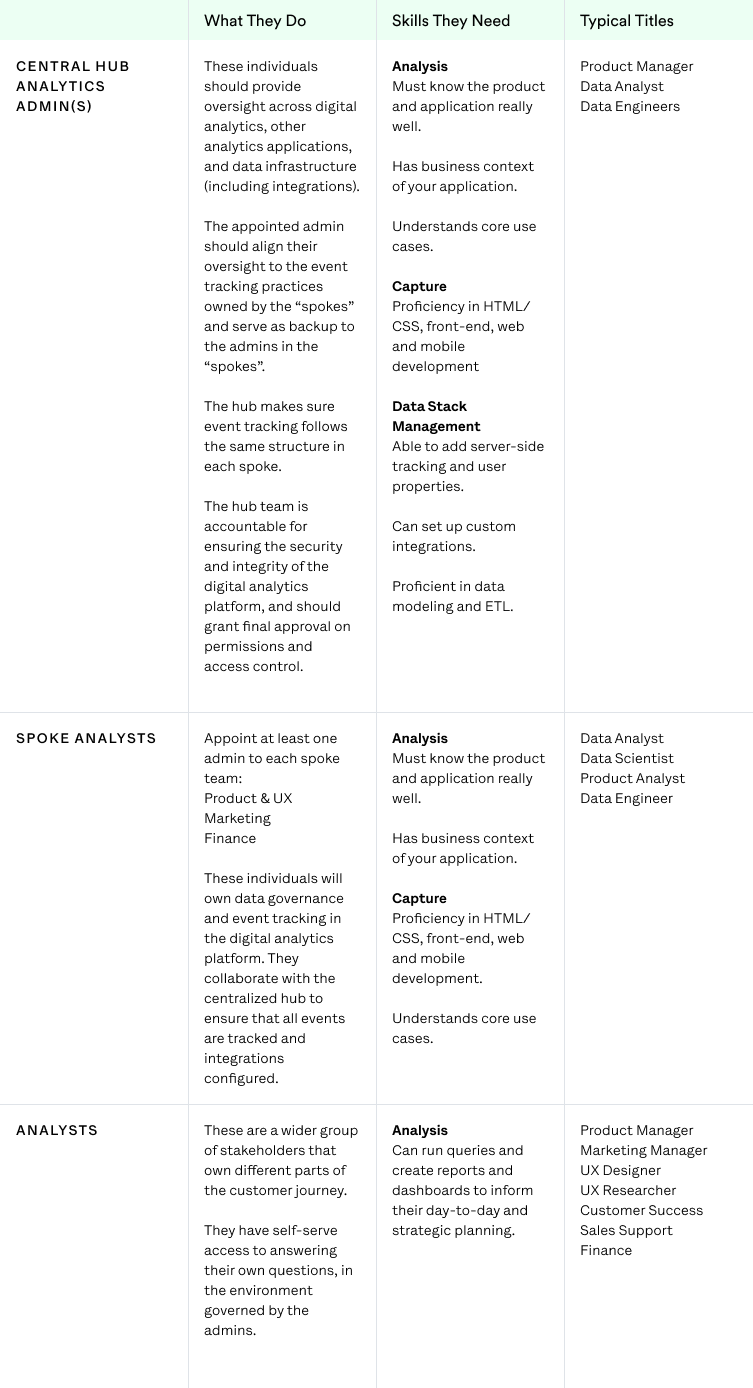
3 - De-centralized model
What is it?
Instead of having one team to handle all of your data analytics needs like the centralized model, a decentralized model means you can distribute your analytics teams across different functions in your business. Each team works independently and takes care of their own analytics.
Now, with a decentralized model, there’s no central oversight or coordination. While that means there’s a risk of data silos and inaccuracy, it does also mean individual business functions can move quickly on their own analytics needs without waiting for approval from others.
Who uses it?
A decentralized model is often used by organizations with a highly decentralized structure, such as large corporations with multiple business units. Start-ups and SMBs also tend to choose this model because they don’t always have the headcount for a centralized data analytics team. However, as these companies scale, the risk of a decentralized model increases, and most businesses end up switching to a centralized or hub and spoke model.
If you’re in the early stages of building your organization and a bottoms-up approach makes the most sense for you, then a decentralized model might be a good fit. Here are some best practices for roles and responsibilities that we recommend:

–
Conclusion
The key to success when it comes to digital analytics is to establish the best team that aligns with your people, process, and technology pillars. Don’t forget to appoint the right people to your analytics team, and carefully manage permissions across your business–no matter the model you choose. While a centralized approach provides the most alignment across your organization, it can also slow down your pace and leave teams answering basic, one-off questions for days.
If balance is more your go-to, then the hub-and-spoke model ensures a clean and trusted dataset with good governance practices. With this model, you can successfully implement, maintain, and optimize digital analytics to support your business needs and goals.
Interested in knowing more about how to leverage your data team to drive needle-moving business impact? Learn more about how to drive data governance, implement analytics change management, and increase data maturity within your organization.